Gauging the Computational Power of the SQMS Center’s High-Quality Superconducting Cavities for Qudit-Based Quantum Algorithms
Numerical simulations suggest that, for a system comprised of a high-Q cavity mode coupled with a transmon, could readily algorithmically control dozens of cavity Fock states when benchmarked using contemporary random sampling tests.
The Science:
Quantum processors comprised of higher-dimensional ‘qudits’ have several potential advantages over the more commonplace two-level qubits. However, it is important to benchmark any potential qudit system in both an algorithmically agnostic fashion as well as in a realistic noisy, ‘full-stack’ setting. This study combines those elements and demonstrates the degree to which the quantum processing units being developed at the SQMS Center perform.
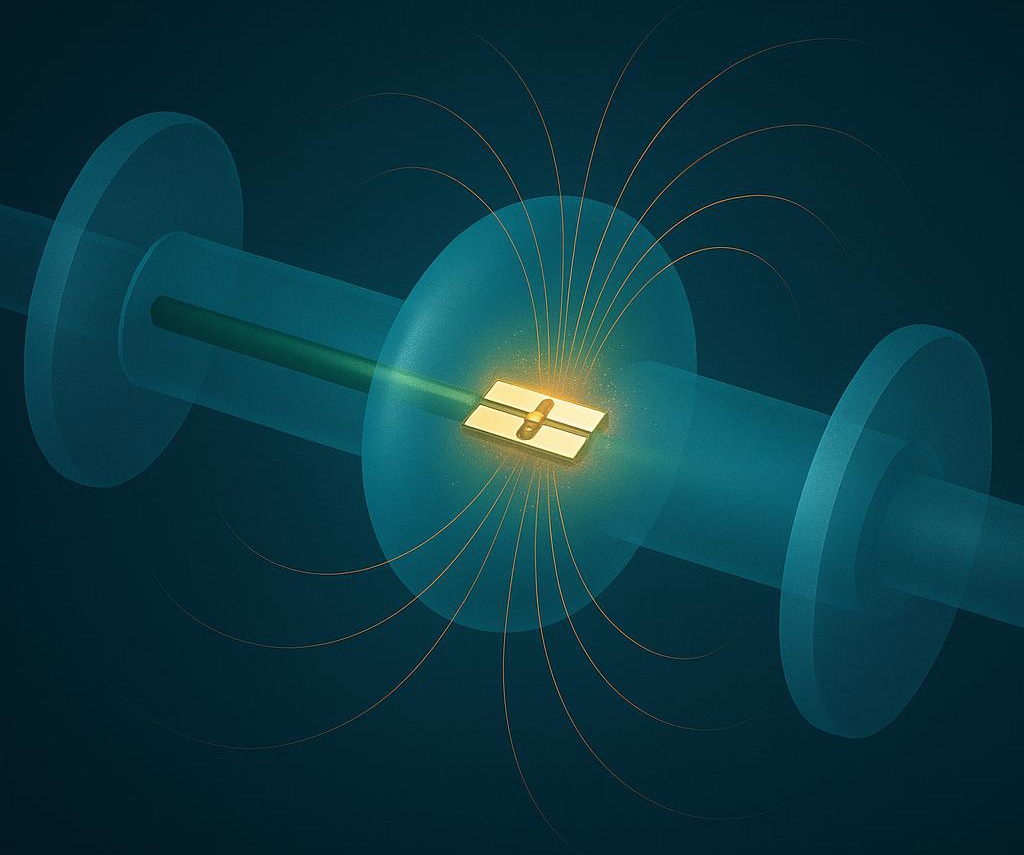
The Impact:
This is the first step towards gauging the performance of the Center’s current and future QPU prototypes compared with QPUs based on other hardware platforms, as well as understanding their advantages and disadvantages.
Summary:
High-quality cavity resonators are excellent resources for storing and processing quantum information in high dimensions when they are connected to ordinary superconducting transmon qubits. As such, it is important to benchmark such systems to both understand their potential as quantum processing units and also improve future hardware prototypes. Here, we develop a full-stack numerical model of just such a system, under both realistic noisy conditions and using a well-known set of universal control operations. Then, we gauge the system’s “algorithmic performance” using adapted versions of popular “sampling” benchmarking tests. Despite the fact that the transmon qubit is often the bottleneck in such systems, our results suggest that one should be able to control a cavity-transmon device to an appreciable degree with relative ease.
Contact:
Nicholas Bornman: nbornman@fnal.gov
Tanay Roy: roytanay@fnal.gov
Focus Areas:
Quantum Algorithms, Simulations and Benchmarking
Institutions:
Fermi National Accelerator Laboratory, Lockheed Martin, Quantum Artificial Intelligence Laboratory, NASA Ames Research Center, USRA Research Institute for Advanced Computer Science
Citation:
Nicholas Bornman, Tanay Roy, Joshua A Job, Namit Anand, Gabriel N Perdue, Silvia Zorzetti and M Sohaib Alam, 2025 Quantum Sci. Technol. 10 025062
https://doi.org/10.1088/2058-9565/adc47c
Acknowledgement:
This material is based upon work supported by the U.S. Department of Energy, Office of Science, National Quantum Information Science Research Centers, Superconducting Quantum Materials and Systems (SQMS) Center under the Contract No. DE-AC02-07CH11359. This document was prepared using the resources of the Fermi National Accelerator Laboratory (Fermilab), a U.S. Department of Energy (DOE), Office of Science, HEP User Facility. Fermilab is managed by Fermi Forward Discovery Group, 1039 LLC under Contract No. 89243024CSC000002 with the U.S. Department of Energy, Office of Science, Office of High Energy Physics. MSA acknowledges support from USRA NASA Academic Mission Services under Contract No. NNA16BD14C with NASA, with this work funded under the NASA-DOE inter-agency agreement SAA2-403602 governing NASA’s work as part of the SQMS Center.